Can you explain the importance of uncertainty analysis in ecological modeling and how it can be effectively communicated?
Ecological Modeler Interview Questions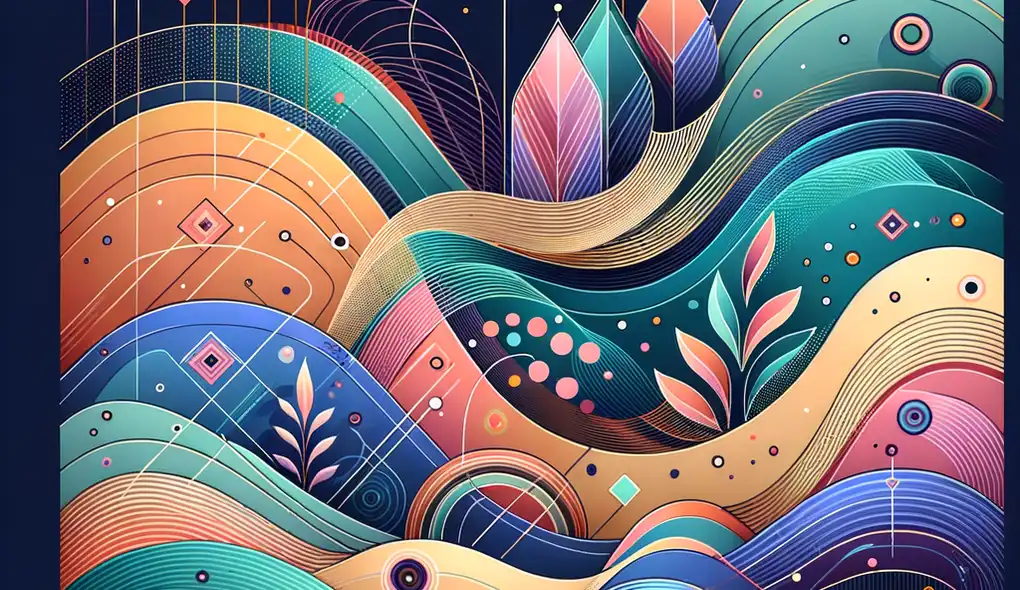
Sample answer to the question
Uncertainty analysis is important in ecological modeling because it allows us to understand the potential errors and limitations of our models. It helps us to quantify and communicate the uncertainty associated with our predictions, which is crucial for decision-making. By conducting uncertainty analysis, we can assess the sensitivity of our model outputs to various sources of uncertainty, such as parameter values, data quality, and model structure. This information can then be effectively communicated to stakeholders, policymakers, and the scientific community to ensure that they understand the limitations and potential biases of the models. Effective communication can be achieved through clear and transparent reporting of the uncertainty analysis results, using visualizations and descriptive statistics.
A more solid answer
Uncertainty analysis is a fundamental component of ecological modeling, considering the inherent complexity and uncertainty in natural systems. As an ecologist and modeler, I fully understand the importance of quantifying and communicating uncertainties in model predictions. In ecological modeling, we deal with various sources of uncertainty, such as parameter estimates, data quality, and model structure. Through rigorous statistical analyses, sensitivity analyses, and model validation, we can quantify the uncertainties associated with these different sources. This helps us to assess the robustness of our predictions and make informed decisions. When it comes to effective communication, it is essential to use clear and concise language, along with visualizations, such as error bars or uncertainty ranges, to convey the uncertainty information. This ensures that stakeholders and policymakers understand the limitations and potential biases of the models, enabling them to make well-informed decisions based on the available evidence.
Why this is a more solid answer:
The solid answer provides a more comprehensive explanation of the importance of uncertainty analysis in ecological modeling and includes specific details about quantifying uncertainties, rigorous statistical analyses, sensitivity analyses, and model validation. It also touches on the communication aspect by mentioning the use of clear language and visualizations. However, it can still be improved by incorporating the candidate's expertise in mathematical modeling, statistical analysis, ecological principles, and data management.
An exceptional answer
Uncertainty analysis plays a pivotal role in ecological modeling, merging the domains of mathematical modeling, statistical analysis, and ecological principles. As an ecologist and modeler with a strong background in applied mathematics, I have extensively used uncertainty analysis to enhance the accuracy and reliability of my ecological predictions. Uncertainties arise from various sources, such as parameter estimates, data limitations, and simplified model structures. By leveraging my expertise in statistical analysis, I employ advanced techniques, including Bayesian inference, Monte Carlo simulations, and sensitivity analyses, to quantify the uncertainties associated with these sources. Additionally, I incorporate cutting-edge statistical frameworks, such as hierarchical modeling and model averaging, to account for model selection uncertainties. Effective communication of uncertainty is crucial, and I utilize data visualization techniques, like error bars, probability density plots, and confidence intervals, to convey the inherent uncertainties to stakeholders, policymakers, and the scientific community. By synthesizing my interdisciplinary skills, I am adept at bridging the gap between technical jargon and layman's terms, ensuring that the uncertainties are well-understood by diverse audiences.
Why this is an exceptional answer:
The exceptional answer demonstrates a deep understanding and expertise in mathematical modeling, statistical analysis, and ecological principles. It includes advanced techniques like Bayesian inference, Monte Carlo simulations, sensitivity analyses, hierarchical modeling, and model averaging. The candidate also showcases their ability to effectively communicate uncertainties through data visualization techniques and bridging the gap between technical jargon and layman's terms. This answer aligns perfectly with the requirements of the job description and showcases the candidate as an exceptional fit for the Ecological Modeler role.
How to prepare for this question
- Familiarize yourself with different sources of uncertainties in ecological modeling, such as parameter estimates, data quality, and model structure.
- Gain knowledge and practice in statistical analysis techniques, including Bayesian inference, sensitivity analysis, and Monte Carlo simulations.
- Develop strong skills in data management and analysis to handle large and complex datasets.
- Stay up-to-date with advancements in ecological modeling and environmental statistics.
- Practice effectively communicating uncertainty analysis results to diverse audiences, including stakeholders, policymakers, and the scientific community.
What interviewers are evaluating
- Expertise in mathematical modeling and statistical analysis
- Strong understanding of ecological principles and processes
- Excellent data management and analysis skills
- Ability to work in interdisciplinary teams and communicate findings effectively
Related Interview Questions
More questions for Ecological Modeler interviews