How do you ensure quality control in your data analysis processes?
Microarray Analyst Interview Questions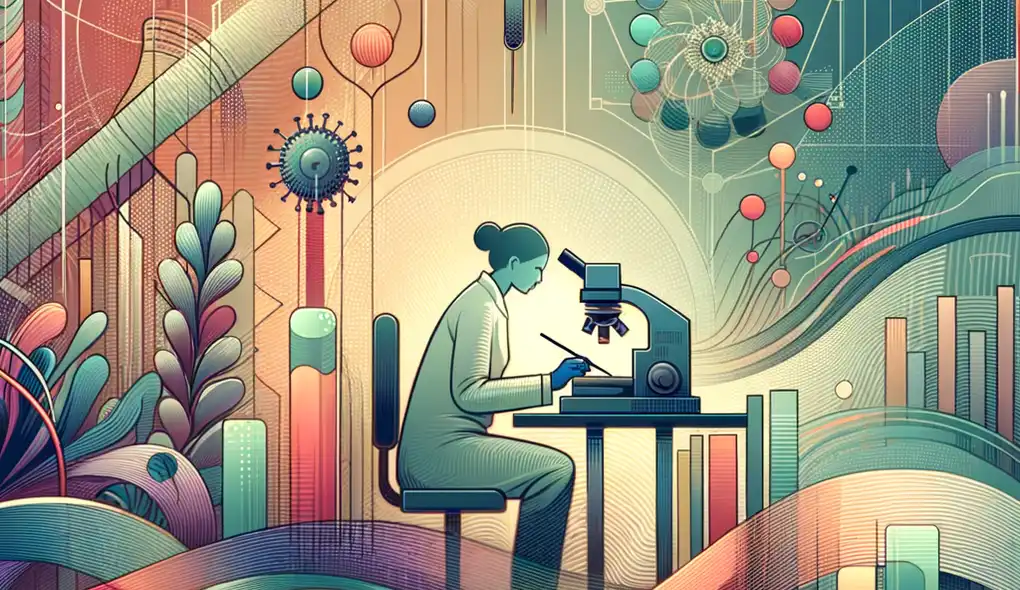
Sample answer to the question
I ensure quality control in my data analysis processes by following a systematic approach. First, I thoroughly review the data to identify any anomalies or errors. Then, I use statistical analysis techniques to analyze the data and identify any outliers or inconsistencies. I also perform validation checks to ensure that the data meets the required standards. Additionally, I collaborate closely with the research team to understand the experimental requirements and ensure that the data analysis aligns with their goals. Finally, I document all my analysis steps and findings to maintain a record of the quality control process.
A more solid answer
Ensuring quality control in my data analysis processes is a top priority for me. I start by carefully examining the data for any inconsistencies or errors. For example, in my previous role, I was analyzing microarray data, and I would meticulously check the gene expression levels to ensure they made sense biologically. To further validate the data, I would perform statistical tests, such as t-tests or ANOVA, to identify any significant differences between groups. Additionally, I would collaborate closely with the research team to understand the experimental design and the specific questions they wanted to answer. This allowed me to tailor my analysis approach and ensure that the data analysis aligned with their goals. Throughout the process, I kept detailed records of my analysis steps and findings, including the parameters used and any data transformations applied. This documentation not only ensured reproducibility but also facilitated transparent communication with stakeholders. Overall, my approach to quality control combines thorough data examination, statistical validation, collaboration, and comprehensive documentation.
Why this is a more solid answer:
The solid answer expands on the basic answer by providing specific examples of how the candidate ensures quality control in data analysis. It also mentions the importance of tailoring the analysis to align with the research team's goals and emphasizes the value of comprehensive documentation. However, it could further improve by discussing additional quality control measures and providing more details on the statistical tests and data validation techniques used.
An exceptional answer
Quality control is an integral part of my data analysis processes, and I employ a multifaceted approach to ensure accuracy and reliability. Firstly, I start by thoroughly understanding the experimental design and goals to identify potential confounding factors and ensure appropriate data preprocessing. For example, in a recent project, I collaborated with biologists to account for batch effects in microarray data by using ComBat normalization. To assess data quality, I adopt a range of statistical techniques, including box plots, QC metrics, and sample clustering, to detect outliers and verify data integrity. As an additional measure, I also employ cross-validation or bootstrapping techniques to evaluate the robustness of my results. Moreover, I maintain a meticulous record of all the steps involved in data analysis, including software versions, parameter settings, and any transformations applied. This documentation allows for transparency and reproducibility. To stay updated with advancements, I actively follow research publications, attend conferences, and engage in discussions with colleagues, ensuring that my methods are aligned with the latest best practices. By meticulously implementing these quality control measures, I can confidently produce reliable analyses that enhance research outcomes.
Why this is an exceptional answer:
The exceptional answer surpasses the solid answer by showcasing the candidate's in-depth knowledge and experience in quality control. It includes specific examples of preprocessing techniques and statistical methods used to ensure data accuracy and integrity. The answer also demonstrates the candidate's proactive approach in staying updated with the latest advancements in the field. However, it could still further improve by discussing the candidate's experience in handling large datasets, as mentioned in the job description, and providing more specific details on how they collaborate with the research team.
How to prepare for this question
- Familiarize yourself with statistical analysis techniques and data visualization tools commonly used in the field of bioinformatics.
- Gain experience in performing quality control checks on large datasets, especially in the context of genomic data analysis.
- Stay updated with the latest advancements in microarray technologies and data analysis methods by reading research publications and attending conferences.
- Develop strong communication and collaboration skills, as working closely with researchers and biologists is crucial in understanding experimental requirements and aligning data analysis with research goals.
- Practice documenting your analysis steps and findings in a clear and organized manner to ensure transparency and reproducibility.
What interviewers are evaluating
- Quality Control
- Data Analysis
- Collaboration
- Documentation
Related Interview Questions
More questions for Microarray Analyst interviews