Staying Ahead of the Curve: Top Data Trends in Education
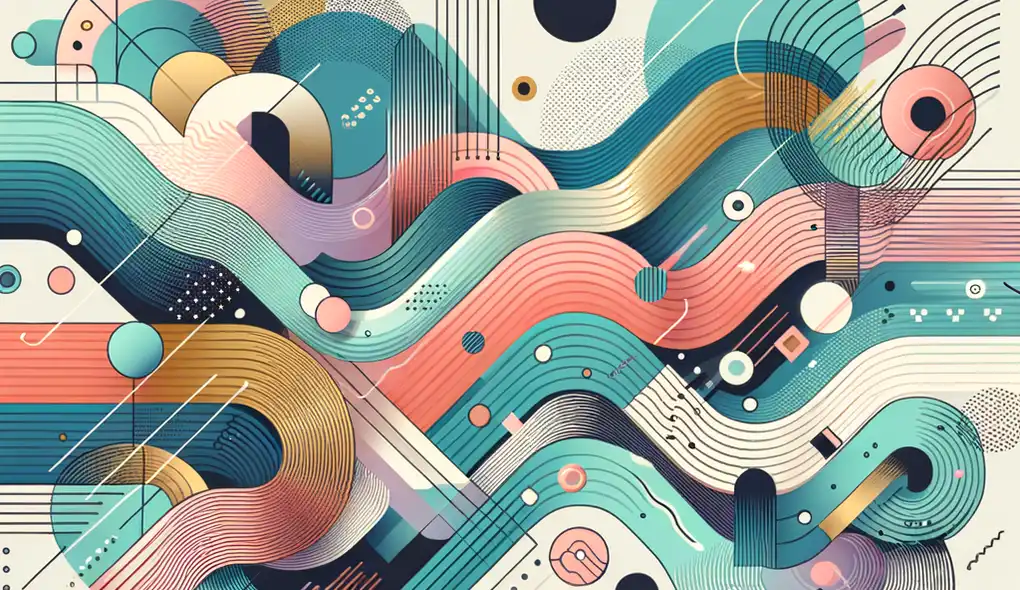
Education, like many other sectors, is undergoing rapid transformation fueled by the avalanche of data being generated in our increasingly digital world. Effective use of educational data can lead to enhanced student outcomes, optimized operational efficiency, and the development of new instructional methodologies. Education Data Analysts play a crucial role in this dynamic environment, turning raw data into actionable insights. As we look at the top data trends in education that analysts should watch, it's important to understand that these trends not only depict current shifts but also foreshadow the future direction of the educational landscape.
Personalized Learning Experiences
One of the most powerful applications of data in education is the creation of personalized learning experiences. With the advent of learning management systems (LMS) and other educational technologies, educators can collect vast amounts of data on student performance and engagement. Analysts can use this data to identify individual learning patterns and customize content to meet each student's unique needs. The trend towards personalized learning is grounded in the belief that education should accommodate diverse learning styles and paces, rather than forcing all students into a one-size-fits-all model.
Predictive analytics plays a significant role in personalized learning. By analyzing historical and real-time data, analysts can predict which students may need additional support or which instructional strategies might be most effective. This forward-looking approach helps educators intervene early and tailor their teaching methods to optimize learning outcomes for every student.
Learning Analytics and Outcome Measurement
Learning analytics refers to the measurement, collection, analysis, and reporting of data about learners and their contexts, for purposes of understanding and optimizing learning and the environments in which it occurs. Outcome measurement, on the other hand, focuses on the end-results of the educational process. Together, these analytical approaches are becoming increasingly prominent as schools and universities seek to validate the effectiveness of their programs.
Data trends reveal a growing emphasis on using learning analytics to track student progress, assess the efficacy of instructional strategies, and inform policy decisions. Such information is invaluable for educators looking to design curricula that align with students' performance dynamics and accreditation standards. Moreover, educational institutions are embracing outcome measurement to prove their value proposition to prospective students, parents, and regulatory bodies.
Integration of Data Sources
The integration of data from various sources is a key trend in the education industry. In the past, student information might exist in silos; today, there's a push to integrate data across systems such as student information systems (SIS), LMS, and customer relationship management (CRM) platforms. This holistic view enables analysts to derive more comprehensive insights into the student journey, from admission to alumni status.
Interoperability standards like xAPI (Experience API) and LTI (Learning Tools Interoperability) facilitate the seamless sharing of data amongst different education technologies. Analysts are now able to track a learner's interactions across various platforms and create a more connected and rich data ecosystem.
AI and Machine Learning in Education Data Analysis
Artificial intelligence (AI) and machine learning (ML) offer transformative potential for education data analysis. These technologies can automate the process of data crunching, identify emerging trends, and even recommend actions. For example, AI-powered chatbots can provide real-time assistance to students, while ML algorithms can sift through vast amounts of data to detect patterns that human analysts may overlook.
The use of AI and ML also extends to educational content creation and assessment. Adaptive learning platforms can dynamically adjust content difficulty and pacing based on student responses, while automated grading systems can provide immediate feedback on assignments, freeing up more time for educators to focus on teaching.
Data Privacy and Ethics
As data becomes a cornerstone of the educational process, issues related to data privacy and the ethical use of student information have come to the forefront. Education Data Analysts must navigate complex regulations, such as the Family Educational Rights and Privacy Act (FERPA) in the United States, and ensure that student data is handled with care.
Amidst rising concerns about data breaches and misuse, the trend is toward securing data and promoting transparency about how student information is collected and used. Analysts have a responsibility to advocate for best practices in data governance, emphasizing the protection of sensitive information, and maintaining the trust of students and their families.
The Growth of EdTech Startups
The EdTech sector has seen a surge in startups aiming to disrupt traditional education with innovative data-driven solutions. These companies leverage data analytics to provide services such as online tutoring, language learning, and test preparation, all of which are tailored to the individual learner.
EdTech startups often adopt a data-centric approach from the outset, building their platforms around the capabilities of data analytics. This positions them well to respond to the changing demands of learners and capitalize on new market opportunities. For Education Data Analysts, the rise of these startups provides a plethora of new datasets to explore and new challenges to tackle.
Conclusion
The educational landscape is evolving at an unprecedented pace, and data is at the core of this transformation. Education Data Analysts need to stay ahead of the curve by keeping abreast of the latest trends and developing the expertise required to harness the power of data for educational advancement. Personalized learning, learning analytics, data integration, AI and ML, data privacy, and the emergence of EdTech startups highlight the expansive terrain Education Data Analysts must navigate. As the field continues to innovate and grow, those who can effectively interpret and leverage educational data will be instrumental in shaping the future of learning.
For educational institutions, the importance of data-informed decision-making cannot be overstated. By understanding and applying the top data trends, Education Data Analysts will play a pivotal role in steering education towards a future where data not only informs but also transforms the educational experience for learners around the globe.
Frequently Asked Questions
Frequently Asked Questions
What is the role of Education Data Analysts in the current educational landscape?
Education Data Analysts play a crucial role in leveraging data to enhance student outcomes, optimize operational efficiency, and develop new instructional methodologies. They are responsible for turning raw educational data into actionable insights that drive decision-making in educational institutions.
How can personalized learning experiences benefit students?
Personalized learning experiences use data to identify individual learning patterns and customize content to meet each student's unique needs. By catering to diverse learning styles and paces, personalized learning can enhance engagement, motivation, and ultimately improve student success rates.
What is the significance of learning analytics and outcome measurement in education?
Learning analytics focuses on understanding and optimizing learning through data analysis, while outcome measurement assesses the effectiveness of educational programs. Together, these approaches help educators track student progress, inform instructional strategies, and demonstrate the value of educational offerings.
How does the integration of data sources impact educational insights?
The integration of data from various sources like student information systems, learning management systems, and CRM platforms provides a holistic view of the student journey. This comprehensive data ecosystem allows analysts to derive deeper insights into student dynamics, from admission to alumni status.
What role do AI and machine learning play in education data analysis?
AI and machine learning technologies automate data analysis processes, identify trends, and offer personalized recommendations. They also support adaptive learning platforms, automated grading systems, and real-time assistance through chatbots, enhancing the efficiency and effectiveness of educational practices.
Why is data privacy and ethics important in education data analysis?
With data privacy concerns on the rise, it is crucial for Education Data Analysts to adhere to regulations like FERPA and prioritize ethical data handling practices. Safeguarding student information, promoting transparency in data usage, and upholding trust are essential responsibilities of analysts in the education sector.
How are EdTech startups reshaping the education industry?
The growth of EdTech startups introduces innovative data-driven solutions into education, such as online tutoring and personalized learning platforms. These startups leverage data analytics to cater to individual learner needs, creating new opportunities for Education Data Analysts to explore diverse datasets and address emerging challenges.
Resources
Further Resources
1. Personalized Learning Experiences
2. Learning Analytics and Outcome Measurement
3. Integration of Data Sources
4. AI and Machine Learning in Education Data Analysis
5. Data Privacy and Ethics
- FERPA: Family Educational Rights and Privacy Act
- International Society of Educational Data Mining (EDM)