Transitioning to Data Science: From Another Career Path
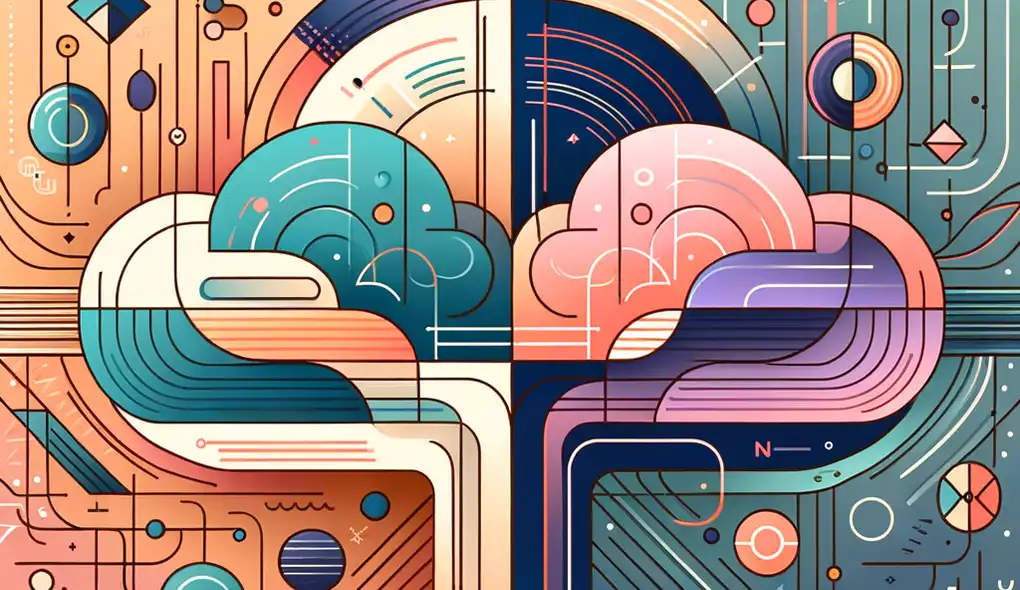
Transitioning to a new career can seem like a daunting endeavor, particularly when it comes to a field as technical and specialized as data science. However, this field is increasingly popular among professionals seeking to leverage their existing knowledge and skills in a market that's hungry for data-driven decision-making. If you are considering a career change and wondering how you might successfully transition to data science from another career path, you're not alone. This article will guide you through the steps and strategies you can employ to make the move both pragmatic and rewarding.
Understanding Data Science
Before diving into the transition to data science, it’s crucial to understand what the field entails. Data science is a multi-disciplinary domain that uses scientific methods, processes, algorithms, and systems to extract insights and knowledge from structured and unstructured data. It combines aspects of mathematics, statistics, computer science, and domain expertise to interpret and communicate data findings in order to solve complex problems.
Assess Your Current Skill Set
The first step in transitioning to data science is to take stock of your current skill set. Professionals with backgrounds in fields such as finance, healthcare, engineering, or even liberal arts, can find aspects of their current expertise that are transferable to data science. For instance:
- Quantitative Analysis: If your current job involves quantitative analysis, you’re already familiar with the kind of logical thinking necessary for data science.
- Programming: Those with programming experience, even if it’s not in data science-specific languages like Python or R, have a foundational skill that’s highly valuable.
- Domain Expertise: Your understanding of a specific industry can be a huge asset, as data science often involves making sense of domain-specific data.
Understanding which skills are transferable is key to knowing where you stand and what you need to learn to become a data scientist.
Fill the Gaps with Education
Once you've identified the skills you need to develop, the next step is to fill those gaps through education. There are numerous ways to acquire data science skills, including:
- Online Courses: Websites like Coursera, Udacity, and edX offer a variety of data science courses, many of which are created by universities or tech companies.
- Bootcamps: Intensive, short-term training programs are designed to teach you data science skills quickly. These often include projects that can serve as portfolio pieces.
- Degree Programs: For a deep dive, consider pursuing a master’s or Ph.D. in data science or a related field.
It's important to choose the educational path that best fits your circumstances, time commitment ability, and learning style.
Build a Portfolio
Developing a portfolio of data science projects is critical. It serves as concrete proof of your skills and can be more telling than any certification. You can start building your portfolio by doing the following:
- Kaggle Competitions: Participate in online data science competitions to showcase your analytical and predictive modeling skills.
- Personal Projects: Work on data science projects based on your interests. These can demonstrate your ability to pose questions and turn data into insights.
- Contributions to Open Source Projects: Being active in the data science community by contributing to open source projects can demonstrate your skills and commitment.
A strong portfolio will often include a variety of projects that demonstrate a range of skills such as data cleaning, analysis, visualization, and machine learning.
Networking and Community Involvement
Networking is an invaluable part of any career change, and transitioning to data science is no exception. Engage with the data science community through:
- Social Media: Platforms like LinkedIn and Twitter are great for connecting with data scientists and discovering industry news.
- Meetups and Conferences: These events can provide opportunities to learn from and network with industry professionals.
- Blogging: Writing about your journey to data science can attract the attention of peers and potential employers.
Active involvement in the data science community not only helps you learn and grow but also increases your visibility to employers.
Gain Real-World Experience
Real-world experience is crucial in proving your worth as a data scientist. Consider the following ways to gain experience:
- Internships: These can provide a foot in the door with a company and valuable hands-on experience.
- Freelancing: Take on freelance projects to not only practice your skills but also earn while you learn.
- Job Transition: If possible, transition into a data-oriented role within your current company or industry before making a full switch to data science. This helps bridge the gap between your current position and your desired career path.
Preparing for the Job Market
As you prepare to enter the data science job market, it’s crucial to tailor your resume and cover letter to highlight your most relevant data science skills and experiences. Be prepared for interviews that may include technical questions, problem-solving exercises, and possibly a review of your portfolio.
In conclusion, transitioning to data science from another career path is certainly feasible with the right approach. It requires assessing and building upon your current skills, investing in education, building a solid portfolio, networking, and gaining practical experience. Data science is an exciting, dynamic field with a wealth of opportunities, and with determination, professionals from various backgrounds can make the move successfully.
Frequently Asked Questions
Frequently Asked Questions
1. Can I transition to data science from a non-technical background?
Yes, it is possible to transition to data science from a non-technical background. Many successful data scientists come from diverse fields such as liberal arts, finance, or healthcare. The key is to identify transferable skills and invest in learning the technical aspects of data science.
2. Do I need a degree in computer science or mathematics to become a data scientist?
While a degree in computer science or mathematics can be beneficial, it is not mandatory to have one to become a data scientist. Many professionals enter the field through alternative education paths such as online courses, bootcamps, or self-study.
3. How long does it take to transition to data science?
The time it takes to transition to data science varies depending on your starting point, the resources available to you, and the intensity of your learning. Some professionals can make the transition in a few months with dedicated full-time study, while others may take a year or more while balancing other commitments.
4. What programming languages should I learn for data science?
Commonly used programming languages in data science include Python, R, and SQL. Python is versatile and widely used in the industry for tasks like data manipulation, analysis, and machine learning. R is popular for statistical analysis and visualization. SQL is essential for working with databases.
5. How important is networking in the data science field?
Networking is crucial in the data science field as it can open up opportunities for collaboration, mentorship, job leads, and knowledge sharing. Engaging with the data science community through events, social media, and online forums can help you stay informed about industry trends and connect with like-minded professionals.
6. Can I pursue data science part-time while working full-time in another field?
Yes, it is possible to pursue data science part-time while working full-time in another field. Many aspiring data scientists start by dedicating evenings and weekends to learning data science skills through online courses or bootcamps. Balancing both commitments requires time management and dedication.
7. What are some common challenges faced by individuals transitioning to data science?
Some common challenges faced by individuals transitioning to data science include the steep learning curve of technical concepts, imposter syndrome, and the pressure to build a competitive portfolio. It's important to stay motivated, seek support from the community, and continuously practice and improve your skills.
8. How can I stay updated with the latest trends and technologies in data science?
To stay updated with the latest trends and technologies in data science, consider following industry experts and thought leaders on social media, subscribing to data science blogs and newsletters, attending conferences and webinars, and actively participating in online communities like GitHub and Stack Overflow.
Resources
Further Resources
For those looking to delve deeper into the world of data science and explore additional resources to aid in their transition, the following comprehensive list is provided:
- Online Courses:
- Bootcamps:
- DataCamp: Specializes in data science and analytics training through interactive learning.
- Springboard: Offers data science bootcamps with mentorship and job guarantee programs.
- Metis: Provides data science bootcamps and corporate training.
- Degree Programs:
- Stanford University - MS in Statistics: Data Science: A comprehensive master's program focusing on data science and statistical methods.
- University of California, Berkeley - Master of Information and Data Science: A fully online program for professionals seeking advanced skills in data science.
- MIT - Statistics and Data Science MicroMasters: Offers a series of online courses in statistics and data science.
- Technical Resources:
- Kaggle: A platform for data science competitions and datasets for practice.
- GitHub: Ideal for sharing and collaborating on data science projects via open source repositories.
- Stack Overflow: A Q&A platform for programming and technical questions related to data science.
- Networking Platforms:
- LinkedIn Groups: Join data science groups to connect with professionals and stay updated on industry trends.
- Meetup: Search for data science meetup groups in your area for networking and knowledge sharing.
- Data Science Central: An online community for data science professionals with forums, blogs, and resources.
- Books and Publications:
- [