Emerging Trends in Data Science: Staying Ahead as a Data Scientist
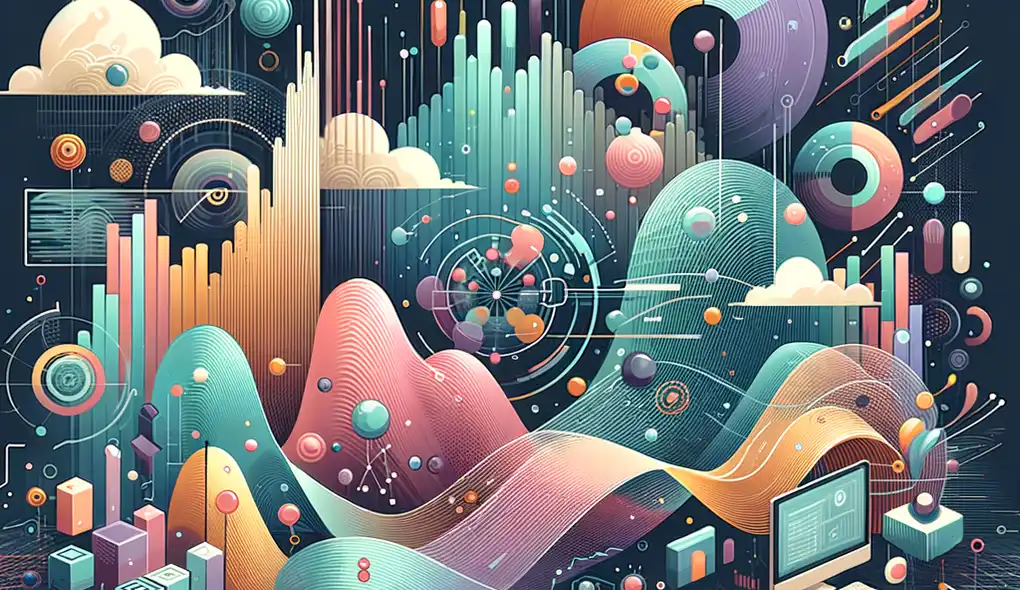
In the dynamic and ever-evolving field of data science, staying current with the latest trends is not just beneficial; it is essential for any professional who wants to remain relevant and successful. As we delve deeper into this golden age of data, emerging trends in data science are revolutionizing how we analyze, interpret, and leverage data across industries. In this comprehensive exploration, we will uncover the cutting-edge developments and how they are shaping the future landscape for aspiring data scientists.
Advancements in Machine Learning and AI
Machine learning (ML) and artificial intelligence (AI) are at the forefront of data science trends, continuously pushing the boundaries of what's possible. No longer confined to science fiction, these technologies are now a driving force behind predictive analytics, natural language processing, and complex decision-making models. Improvements in algorithms, paired with enhanced computational power, are leading to more accurate and swift predictions, making real-time data processing a reality for many businesses.
Enhanced Data Literacy
As data becomes more integral to decision-making, a trend towards enhanced data literacy is emerging. Companies are recognizing the importance of empowering their workforce with the skills to collect, analyze, and interpret data. This democratization of data science skills is leading to a culture shift where data fluency is becoming just as important as basic computer literacy was in the past. Aspiring data scientists should now focus on not only technical skills but also on communicating insights effectively to stakeholders.
The Rise of Data Engineering
With a proliferation of data, the role of the data engineer has become more crucial than ever. Data engineering focuses on the architecture and infrastructure necessary for gathering, storing, and processing large datasets. As businesses demand more sophisticated data pipelines and real-time streaming capabilities, expertise in data engineering is increasingly sought after. For those looking to break into data science, understanding the foundations of data engineering can provide a significant edge.
Quantum Computing's Impact on Data Science
Quantum computing, though still in its nascent stage, is positioning itself as a revolutionary force in data science. With its ability to process complex computations exponentially faster than traditional computers, quantum computing is anticipated to unlock new potentials in data modeling, optimization, and encryption. Aspiring data scientists who are familiar with the basics of quantum mechanics and its application to data science may find themselves at the forefront of an industry transformation.
The Expansion of IoT and Edge Computing
Internet of Things (IoT) devices are becoming ubiquitous, and with them, edge computing is rising as a significant trend. Edge computing refers to processing data where it's generated—at the device level—rather than relying on distant servers. This reduces latency, increases the speed of insights obtained from data analytics, and supports more intelligent and autonomous device behavior. Understanding IoT and edge computing will be beneficial for data scientists looking to work on cutting-edge predictive maintenance, smart cities, health tech, and more.
Ethical AI and Responsible Data Practices
As the capabilities of AI grow, so too does the responsibility to use it ethically. Data scientists must now grapple with the societal impacts of their models and the data they use. This has given rise to an increased emphasis on ethical AI practices and responsible data management, focusing on fairness, privacy, transparency, and accountability. Aspiring data scientists will need to balance the power of data with the ethical implications of their work.
Cloud-Native Technologies
The reliance on cloud computing has ushered in the age of cloud-native technologies, including serverless architectures and containerization. These advancements provide data scientists with scalable, efficient, and flexible platforms for deploying models and conducting analyses. Familiarity with cloud services and platforms such as AWS, Azure, and Google Cloud Platform can greatly enhance a data scientist's toolkit.
AutoML and Democratized Data Science
Automated machine learning (AutoML) is democratizing data science, enabling users without an extensive background in ML to develop models and facilitate analysis. This lowers the entry barrier for new data scientists and broadens the potential for insights across different roles within an organization. However, an understanding of the principles behind the models remains crucial for ensuring that conclusions drawn are valid and trustworthy.
The Emphasis on Soft Skills
Technical expertise is pivotal, but the demand for soft skills such as critical thinking, problem-solving, and especially communication, is increasing. Data scientists must articulate complex findings to non-technical audiences. Therefore, refining these soft skills will complement technical know-how and make data science insights more actionable and impactful.
Specialization in the Field
Finally, as the field of data science broadens, we are witnessing a trend towards specialization. Rather than being a jack-of-all-trades, data scientists are deepening their expertise in specific areas such as healthcare, finance, or geospatial analysis. This specialization allows for a more in-depth understanding of industry-specific challenges and opportunities, enhancing the impact of data science in those sectors.
As we look forward to the future of data science, it is clear that the field is poised for even greater heights. The trends discussed here are just a snapshot of the ongoing evolution. For aspiring data scientists, staying ahead means continually learning, adapting, and applying these trends in practical, ethically responsible ways. By doing so, they will not only shape their own future but also the future of their respective industries and the global landscape of data-driven decision-making.
Frequently Asked Questions
Frequently Asked Questions
What are the key trends in data science that aspiring data scientists should be aware of?
Aspiring data scientists should be aware of key trends such as advancements in machine learning and AI, enhanced data literacy, the rise of data engineering, the impact of quantum computing, expansion of IoT and edge computing, ethical AI practices, cloud-native technologies, AutoML, emphasis on soft skills, and specialization in the field.
How can data scientists stay current with emerging trends in the field?
Data scientists can stay current by regularly attending conferences, workshops, and webinars focused on data science trends. They can also join online communities, participate in data science challenges, and constantly upskill through courses and certifications.
What are the essential skills for a successful data scientist?
Essential skills for a successful data scientist include proficiency in programming languages like Python and R, strong understanding of statistics and mathematics, ability to work with large datasets, knowledge of machine learning algorithms, data visualization skills, and effective communication skills.
How important is ethical AI in data science?
Ethical AI is crucial in data science as it ensures that models and data practices are fair, transparent, and accountable. Data scientists need to consider the ethical implications of their work to build trust, prevent bias, and uphold societal values.
Is specialization necessary in the field of data science?
Specialization in data science allows professionals to deepen their expertise in specific domains like healthcare, finance, or geospatial analysis. While not mandatory, specialization can enhance the impact of data science by addressing industry-specific challenges more effectively.
What role does cloud computing play in data science?
Cloud computing provides data scientists with scalable and flexible infrastructure for storing, processing, and analyzing large datasets. Platforms like AWS, Azure, and Google Cloud offer tools and services that streamline data science workflows and enable collaboration in a cloud-native environment.
Resources
Further Resources
For readers interested in delving deeper into the emerging trends in data science and staying ahead as a data scientist, the following resources provide additional insights, tutorials, and guidance:
- Towards Data Science: A platform that offers a wide range of articles on data science trends, techniques, and tutorials.
- Kaggle: A community of data scientists and machine learning practitioners where users can access datasets, participate in competitions, and learn from others.
- DataCamp: An online platform offering courses on data science, machine learning, and data engineering for all skill levels.
- AI Ethics: Resources from the Rockefeller Foundation on ethical AI practices and guidelines.
- MIT Technology Review: Stay updated with the latest news and insights on technology, including data science trends.
- Coursera: Offers courses from universities and institutions on various data science topics, including machine learning, data engineering, and AI.
- Books on Data Science: Explore a curated list of essential books on data science recommended by industry experts.
- Data Science Central: A community-driven platform for data science professionals to engage, learn, and share resources.
- OpenAI: Learn about cutting-edge AI research and developments from OpenAI, a leading AI research laboratory.
- LinkedIn Learning: Access data science courses and tutorials on LinkedIn Learning to enhance your skills and stay updated on industry trends.
These resources cover a wide spectrum of data science topics, from foundational knowledge to advanced techniques, and can serve as valuable tools for professionals looking to advance their careers in data science.