Advanced Data Analysis Techniques in Healthcare You Should Know
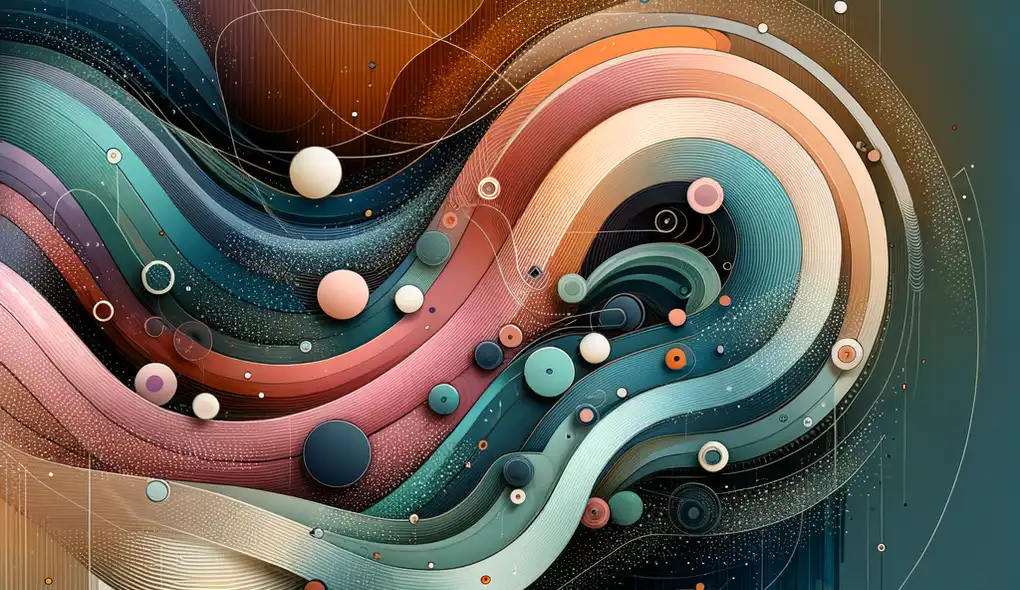
The evolution of data science and analytics has had a profound impact on the healthcare industry, transforming how care is delivered, health outcomes are predicted, and medical research is conducted. With an ever-growing volume of data generated by electronic health records (EHRs), wearable devices, and other medical technologies, the capability to leverage advanced data analysis techniques has become indispensable for healthcare professionals. In this article, we dive into several state-of-the-art data analysis methods that are reshaping healthcare analytics, providing deeper insights, and enhancing patient care.
Predictive Modeling
Predictive modeling is at the forefront of data analytics in healthcare. Leveraging various statistical techniques and machine learning algorithms, predictive models can forecast patient outcomes, readmission risks, and potential side effects of treatment. By analyzing historical data, these models identify patterns and correlations that can infer the likelihood of future events. This technique enables healthcare providers to intervene proactively, tailor treatment plans, and ultimately improve the patient journey.
Some of the most prominent predictive modeling techniques in healthcare include logistic regression, decision trees, random forests, and neural networks. Each technique has its strengths and is chosen based on the nature of the prediction required and the characteristics of the dataset. For instance, logistic regression is widely used for binary outcomes such as disease presence or absence, whereas neural networks, with their deep learning capabilities, are excellent for complex pattern recognition tasks.
Natural Language Processing (NLP)
Natural Language Processing (NLP) opens up vast opportunities in healthcare by enabling the extraction of valuable insights from unstructured text data such as clinical notes, patient feedback, and medical literature. Advanced NLP techniques involve sentiment analysis, entity recognition, and topic modeling, which can facilitate better patient engagement, enhance clinical decision-making, and accelerate research discovery. By automating the interpretation of textual information, NLP helps in reducing manual labor and minimizing errors associated with manual data entry.
Time Series Analysis
Time series analysis is crucial in monitoring patient health over time, understanding disease progression, and evaluating the effect of interventions. Techniques such as ARIMA (AutoRegressive Integrated Moving Average) and LSTM (Long Short-Term Memory) networks help to capture trends, cycles, and irregular patterns in healthcare data. This analysis aids in identifying seasonal outbreaks of diseases, forecasting demands for hospital resources, and understanding long-term patient outcomes, which are essential for strategic planning in healthcare facilities.
Image Analysis and Computer Vision
Advanced imaging techniques combined with machine learning algorithms have revolutionized medical diagnostics. Techniques like convolutional neural networks (CNNs) are adept at processing visual data and have been employed to detect abnormalities in radiological images, evaluate histopathological slides, and screen for retinal diseases. Image analysis and computer vision hold the potential to automate and augment the interpretation of medical images, allowing for faster and more accurate diagnoses.
Genomic Data Analysis
The field of genomics has expanded the horizon of personalized medicine by analyzing an individual's genetic makeup. Bioinformatics and computational genetics involve sophisticated algorithms for mapping, sequencing, and comparing genetic data. These analyses can identify genetic markers linked to diseases, predict response to medication, and personalize treatment strategies. Techniques like GWAS (Genome-Wide Association Studies) and machine learning-based predictive models are transforming our understanding of the genetic underpinnings of health and diseases.
Prescriptive Analytics
Building on predictive analytics, prescriptive analytics goes a step further by not only forecasting outcomes but also suggesting actions to achieve desired goals. Using optimization, simulation, and decision-analysis techniques, prescriptive analytics can help healthcare providers make data-driven decisions about patient care plans, resource allocation, and policy-making.
Network Analysis
Network analysis explores how different entities such as genes, proteins, diseases, and patients are interconnected. This analysis is useful for understanding complex relationships in large datasets, such as the interactions within biological systems or the spread of infectious diseases. By mapping out these connections, healthcare professionals can identify key drivers of diseases, potential drug targets, and social determinants of health.
Conclusion
Advanced data analysis techniques play a critical role in unlocking the potential of big data in healthcare. As healthcare organizations continue to grow their data capabilities, the adoption of these cutting-edge methods will be vital in developing a more predictive, personalized, and efficient healthcare system. While these techniques offer remarkable opportunities for innovation, it is essential to approach them with an understanding of their complexity and the ethical considerations involved in handling sensitive health data. For data-savvy healthcare professionals, staying up-to-date with these techniques will be crucial for advancing patient care and leading the next wave of healthcare transformation.
Frequently Asked Questions
When it comes to advanced data analysis techniques in healthcare, it's common for professionals to have questions about the best practices, implementation challenges, and real-world applications. In this Frequently Asked Questions section, we address some of the key queries that arise in the realm of healthcare analytics.
Frequently Asked Questions
1. What are the main challenges in implementing predictive modeling in healthcare?
Implementing predictive modeling in healthcare comes with various challenges, including the need for high-quality data, interpretation of complex algorithms, and ensuring regulatory compliance. Healthcare professionals may also face resistance to change and skepticism about the accuracy of predictive models. Overcoming these challenges requires collaboration between data scientists, clinicians, and IT specialists to ensure the successful integration of predictive modeling into clinical practice.
2. How can Natural Language Processing (NLP) improve patient outcomes?
NLP can improve patient outcomes by enabling healthcare providers to extract valuable insights from unstructured text data, such as patient records and clinical notes. By automating the analysis of textual information, NLP can enhance clinical decision-making, identify trends in patient feedback, and personalize treatment plans. This technology has the potential to streamline data analysis processes, leading to better patient outcomes and more efficient healthcare delivery.
3. What is the significance of Time Series Analysis in healthcare?
Time Series Analysis is significant in healthcare for monitoring patient health trends, predicting disease outbreaks, and optimizing resource allocation. By analyzing temporal data, healthcare professionals can identify patterns, seasonal variations, and long-term trends that impact patient care. Time Series Analysis enables proactive decision-making, risk assessment, and strategic planning, making it a valuable tool for healthcare organizations seeking to improve patient outcomes and operational efficiency.
4. How do advanced imaging techniques benefit medical diagnostics?
Advanced imaging techniques, combined with machine learning algorithms, enhance medical diagnostics by enabling the detection of subtle abnormalities and providing quantitative insights from visual data. Technologies like convolutional neural networks (CNNs) facilitate automated image analysis, leading to faster and more accurate diagnoses. By leveraging image analysis and computer vision, healthcare providers can improve precision in diagnosis, treatment planning, and patient monitoring, ultimately enhancing the quality of care.
5. What role does Genomic Data Analysis play in personalized medicine?
Genomic Data Analysis plays a crucial role in personalized medicine by analyzing genetic information to tailor treatment strategies and predict individual responses to medications. By identifying genetic markers associated with diseases, healthcare providers can offer personalized interventions that target the underlying genetic factors. Genomic Data Analysis also contributes to the advancement of precision medicine by guiding therapeutic decisions and preventive measures based on an individual's genetic makeup.
6. How can prescriptive analytics support data-driven decision-making in healthcare?
Prescriptive analytics supports data-driven decision-making by providing actionable insights and recommendations based on predictive models and optimization techniques. Healthcare providers can use prescriptive analytics to optimize resource allocation, improve patient outcomes, and enhance operational efficiency. By leveraging prescriptive analytics, organizations can make informed decisions that lead to better patient care, cost savings, and strategic planning.
7. What are the key considerations for conducting network analysis in healthcare?
When conducting network analysis in healthcare, it is essential to consider data quality, network structure, and the relevance of connections within the system. Healthcare professionals should prioritize data accuracy, integrity, and security to ensure reliable network analysis outcomes. Understanding the underlying relationships between different entities in healthcare networks can help identify critical pathways, optimize interventions, and improve the overall efficiency of healthcare delivery.
By addressing these frequently asked questions, healthcare professionals can gain a deeper understanding of the nuances and applications of advanced data analysis techniques in healthcare, enabling them to make informed decisions and drive innovation in patient care and healthcare management.
Resources
Further Resources
For readers interested in delving deeper into advanced data analysis techniques in healthcare, the following resources provide valuable insights and learning opportunities:
- Predictive Modeling
- Natural Language Processing (NLP)
- Time Series Analysis
- Image Analysis and Computer Vision
- Genomic Data Analysis
- Prescriptive Analytics
- Network Analysis