Back to Data Science ManagerEffective Leadership in Data Science: A Manager's Guide
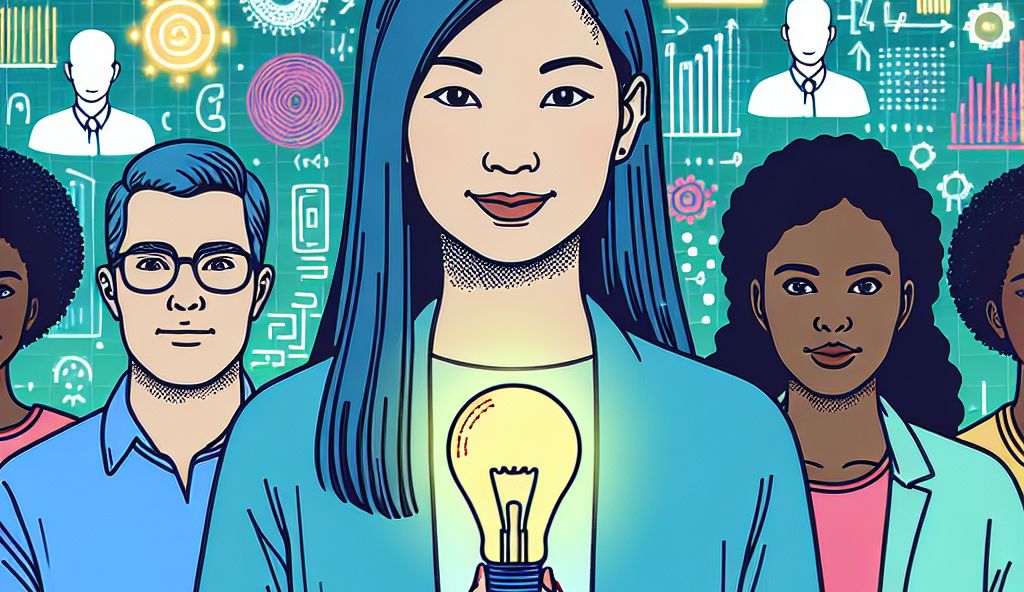
The integration of data science into various aspects of business operations and decision-making has led to a significant transformation in the corporate world. With its power to turn big data into insightful analytics, data science offers companies a competitive edge. However, the success of data science initiatives heavily relies on effective leadership. As a manager in the data science field, it's crucial to understand what makes leadership effective to guide your team toward achieving outstanding results. This comprehensive guide explores the leadership styles, qualities, and strategies that are most conducive to success in the realm of data science.
Before delving into leadership, it's important to understand the ecosystem that data science operates within. This field encompasses a variety of disciplines, including statistics, machine learning, artificial intelligence, and big data processing. The role of a Data Science Manager is to oversee projects that draw upon these areas, ensuring that their team can harness the full potential of the data. An effective leader must therefore be conversant with current technological trends, data privacy standards, and ethical considerations. This forms the basis of making informed decisions and fostering an innovative environment.
There are several leadership styles that can be adopted within the realm of data science, each with its own set of advantages and challenges:
- Transformational Leadership: This style involves inspiring and motivating team members to exceed their existing capabilities and expectations. Transformational leaders focus on fostering a proactive culture of innovation and continuous improvement, aligning organizational goals with personal development.
- Democratic Leadership: Leaders who follow this style actively encourage team participation in decision-making processes. It fosters a sense of ownership and responsibility among team members, which can be especially beneficial in a data-driven environment where collaboration is key.
- Autocratic Leadership: This more directive approach can be useful in data science when decisions need to be made swiftly and when the leader possesses the most knowledge on the subject. However, it can stifle creativity and team morale if overused.
- Servant Leadership: In this approach, the leader's primary role is to serve the team, helping to remove obstacles and providing the resources needed for them to succeed. It can lead to high levels of team engagement and job satisfaction.
Being an effective leader in the data science field requires a unique blend of technical prowess and soft skills, including:
- Technical Competence: A strong understanding of data science methodologies and tools is essential. Staying updated with the latest industry developments allows you to make better strategic choices and lead your team effectively.
- Communication Skills: Clear and concise communication is vital, especially when explaining complex data science concepts to stakeholders who may not have a technical background.
- Strategic Thinking: A data science manager must think strategically to align data science projects with business objectives.
- Emotional Intelligence: The ability to understand and manage both your own emotions and those of your team is critical in leading a diverse team of professionals.
With the right strategies in place, you can strengthen your leadership and drive your team to success. Here are some key strategies to consider:
- Empower Your Team: Give team members autonomy to explore and innovate, while providing guidance and support. Encourage them to attend workshops, conferences, and further their education to stay on top of industry trends.
- Promote Collaboration: Foster a collaborative culture where data scientists, engineers, analysts, and business units work together seamlessly. Collaboration can help unlock new insights and drive innovative solutions.
- Make Data-driven Decisions: As a leader in data science, prioritize evidence over intuition. Implement systems that facilitate the continuous, real-time analysis of data to inform your decisions.
- Recognize and Cultivate Talent: Identify the individual strengths of each team member and provide opportunities to develop those skills. Recognition of efforts and achievements also motivates individuals to excel.
- Stay Agile: Be adaptable in your leadership approach and open to change. Data science is a rapidly evolving field, and an agile mindset will help your team pivot effectively when needed.
- Create a Positive Work Culture: A positive work culture that encourages experimentation without the fear of failure is key to innovation in data science.
- Align Projects with Business Goals: Communicate convincingly the relevance of data science projects to overarching business goals to maintain stakeholder support and ensure your team's work has impact.
Effective leadership in data science is not solely about managing data or understanding algorithms. It's about creating a vision, inspiring your team, and fostering an environment where innovation thrives. By adapting your leadership style to the needs of your team, communicating effectively, making data-driven decisions, and continuously seeking to improve both your technical and managerial skills, you can excel as a Data Science Manager. Remember, the best leaders are those who constantly learn, adapt, and enable their teams to reach new heights.
In data science, key leadership styles include Transformational Leadership, Democratic Leadership, Autocratic Leadership, and Servant Leadership. Each style has its own benefits and challenges, impacting team dynamics and project outcomes.
Technical competence is crucial for a Data Science Manager as it forms the foundation for making informed decisions, guiding the team effectively, and staying abreast of industry advancements. A strong understanding of data science methodologies and tools is essential.
Emotional intelligence plays a vital role in effective leadership as it enables managers to understand and manage emotions, resolve conflicts, build strong relationships, and nurture a positive work environment. It is especially important when leading diverse teams in data science.
Data Science Managers can promote a culture of innovation by empowering their team members to explore new ideas, providing resources for professional development, encouraging collaboration across different roles, and creating a positive work culture that values experimentation.
Aligning data science projects with business goals is essential to ensure that the work contributes to the overall objectives of the organization, maintains stakeholder support, and demonstrates the impact of data-driven insights on key business metrics.
For additional insights into effective leadership in the field of data science, consider exploring the following resources:
- Books:
- The Leadership Challenge by James M. Kouzes and Barry Z. Posner
- Leaders Eat Last: Why Some Teams Pull Together and Others Don't by Simon Sinek
- Online Courses:
- Coursera offers courses on Leadership and Data Science Management that can enhance your leadership skills in the field.
- Udemy provides a variety of courses on Effective Communication and Strategic Thinking tailored for data science managers.
- Webinars and Workshops:
- Stay updated on the latest trends in data science leadership through webinars hosted by organizations like Data Science Central and Dataconomy.
- Professional Organizations:
- Join professional organizations such as the Data Science Association or the Data and Analytics Leaders Network for networking opportunities and valuable resources on leadership.
- Blogs and Articles:
- Explore blogs like Towards Data Science and KDnuggets for in-depth articles on leadership strategies specific to the data science industry.
- Podcasts:
- Tune in to podcasts such as Data Skeptic and The Leadership Podcast for discussions on data-driven decision-making and effective leadership techniques.
- LinkedIn Learning:
- Access a wide range of courses and videos on leadership styles, emotional intelligence, and team management suitable for data science professionals on LinkedIn Learning.
- Industry Conferences:
- Attend conferences like the Data Science Leaders Summit and Strata Data Conference to network with industry experts and gain valuable insights into leading data science teams effectively.
By exploring these resources, you can further enhance your leadership skills and stay abreast of the evolving landscape of data science management.