Why Quality Data Analysis is an In-Demand Skillset
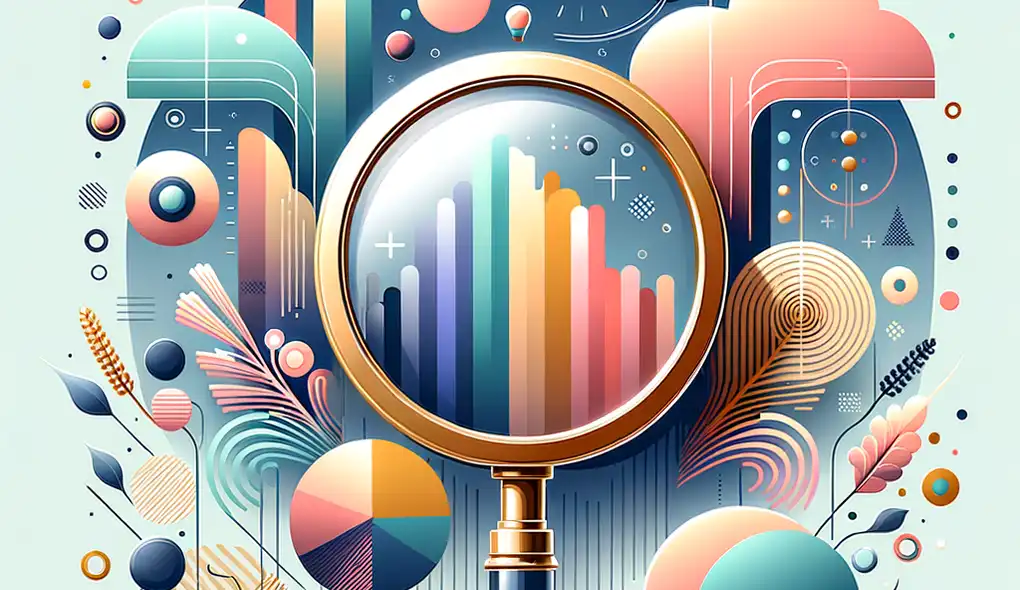
In the digital age, data is everywhere. Collecting it is one thing; making sense of it is entirely another. It's the latter where quality data analysis plays a pivotal role. The demand for quality data analysis is higher than ever, and it's not difficult to see why. The skillset required to interpret, dissect, and draw meaningful insights from data is increasingly becoming a game-changer across various industries. In this article, we dive deep into the reasons behind this growing demand and provide guidance on how to position yourself favorably in the job market.
The Value of Data in Decision-Making
Data analysis is fundamental to the decision-making process. Organizations rely on data to make informed decisions that can lead to improved performance, innovation, and competitiveness. Quality data analysis transforms raw data into actionable insights. This not only helps organizations understand their current state but also predict future trends and customer behaviors.
Take the healthcare industry, for instance. Here, data analysis leads to better patient outcomes by identifying patterns and trends in patient data. The same can be said for finance, where analysts can predict market trends and guide investment strategies.
Industry-wide Adoption
The ubiquity of data has meant that its analysis is not confined to traditionally data-heavy sectors like finance or tech. Sectors such as agriculture, education, and even sports are now employing data analysts to optimize their operations and strategies. This broad adoption signifies that proficiency in data analysis is an asset regardless of one's field of expertise.
The Role of Big Data and AI
The Big Data revolution has compounded the value of data analysts. The sheer volume, velocity, and variety of data generated today require sophisticated analysis that goes beyond traditional methods. Artificial intelligence (AI) and machine learning (ML) have become integral tools for analysts. They have the power to automate tasks, detect complex patterns, and improve the accuracy of predictions in a way that humans alone cannot.
Business Intelligence and Reporting
Quality data analysis is also essential for effective business intelligence (BI). BI tools and systems depend on the robust analysis of data to provide real-time reporting and dashboards. These insights enable leaders to monitor the health of their business and make tactical decisions swiftly.
The Skills Gap
Despite its importance, there is a notable skills gap in the market. Many organizations struggle to find qualified data analysts who can not only crunch numbers but also interpret them in a business context. This mismatch creates a significant opportunity for professionals who can bridge this gap.
Preparing for a Career in Data Analysis
Those looking to enter or advance in the field of data analysis need a blend of technical and soft skills. A stronghold on statistical methods, proficiency in data analysis tools (such as SQL, Python, or R), and an understanding of AI and ML are critical. Equally important is the ability to communicate findings to a non-technical audience effectively.
Educational Pathways
Science, technology, engineering, and mathematics (STEM) fields traditionally serve as a pipeline for data analysts. However, many analysts come from diverse backgrounds, including business, economics, and even humanities. With the right training and certifications, such as a degree in data science or relevant boot camps, individuals from various academic backgrounds can enter this field.
Data Analyst vs. Data Scientist
While the roles might seem similar, data scientists typically work on more complex problems and may develop new algorithms for data analysis. Data analysts, on the other hand, focus more on the interpretation and application of existing data to business questions.
Certification and Continuous Learning
Certifications can be a gateway for professionals looking to prove their skills or transition into data analysis. Continuous learning is essential, as the field is dynamic and tools and methodologies are continually evolving.
The Future of Data Analysis
Looking to the future, the demand for data analysts is expected to grow. Automation and AI might change the nature of the work, but they will not eliminate the need for human insight. On the contrary, they will elevate the role of data analysts who can work alongside advanced technologies to provide deeper insights.
Conclusion
In summary, quality data analysis is increasingly recognized as a critical skillset in today’s data-driven world. It's a ticket to many opportunities across industries, offering a path to impactful and strategic roles within organizations. As we generate more data than ever before, the mastery of this skillset not only makes one employable but indispensable. For those looking to make a mark in the employment industry through data analysis, the first step is embracing the opportunity for lifelong learning and remaining adaptable to the ever-changing digital landscape.
Frequently Asked Questions
Frequently Asked Questions about Data Analysis
Q: What is data analysis, and why is it important in today's job market?
A: Data analysis is the process of inspecting, cleansing, transforming, and modeling data to uncover useful information and support decision-making. In today's job market, data analysis is crucial as it helps organizations make informed decisions, understand trends, and predict outcomes.
Q: What skills are needed to excel in data analysis roles?
A: To excel in data analysis roles, individuals need a strong foundation in statistical methods, proficiency in data analysis tools like SQL, Python, or R, knowledge of AI and ML, and effective communication skills to translate technical findings for non-technical audiences.
Q: How can someone transition into a data analysis career from a different field?
A: Transitioning into a data analysis career from a different field is possible through acquiring relevant certifications, taking online courses, participating in boot camps, and showcasing transferable skills such as problem-solving, critical thinking, and attention to detail.
Q: What is the difference between a data analyst and a data scientist?
A: While both roles involve working with data, data scientists typically handle more complex problems and may develop new algorithms, whereas data analysts focus on interpreting and applying existing data to derive insights for business decisions.
Q: How can continuous learning and certification benefit a data analyst's career?
A: Continuous learning and certifications can elevate a data analyst's career by keeping them updated on the latest tools and methodologies, showcasing their expertise to potential employers, and opening up opportunities for advancement and specialization.
Q: What is the future outlook for data analysis professionals?
A: The future outlook for data analysis professionals is promising, with the demand for skilled data analysts expected to continue growing. While automation and AI may alter the nature of the work, human insight and analytical skills will remain essential in providing meaningful interpretations of data.
For more information and resources on data analysis, certifications, and career pathways, check out the following links:
Resources
Further Resources
For those interested in delving deeper into the world of data analysis and advancing their skills in this in-demand field, here are some valuable resources to explore:
- Online Courses and Certifications:
- Books on Data Analysis:
- “Data Science for Business” by Foster Provost and Tom Fawcett
- “Practical Statistics for Data Scientists” by Peter Bruce and Andrew Bruce
- Professional Networking and Forums:
- Join LinkedIn groups like “Data Science & Analytics” for networking and industry updates
- Participate in forums like Kaggle and Data Science Central for discussions and shared learning
- Data Visualization Tools:
- Explore tools like Tableau, Power BI, and Google Data Studio for enhancing data visualization skills
- AI and Machine Learning Resources:
- Enroll in machine learning courses on platforms like Udacity and Kaggle
- Follow AI thought leaders on platforms like Medium and Towards Data Science
- Industry Conferences and Webinars:
- Attend data science conferences like Data Science Summit and Strata Data Conference
- Stay updated on the latest trends through webinars by industry experts
- Data Analysis Blogs and Publications:
- Read blogs like Data Science Central and KDnuggets for insights and tutorials
- Subscribe to publications like Harvard Business Review and MIT Technology Review for data analysis articles
- Coding Practice Platforms:
- Sharpen your coding skills on platforms like LeetCode and HackerRank
By leveraging these resources and continuously investing in your learning journey, you can stay ahead in the dynamic field of data analysis and excel in your career pursuits.