Advanced Topics in Quantitative Research: What You Need to Know
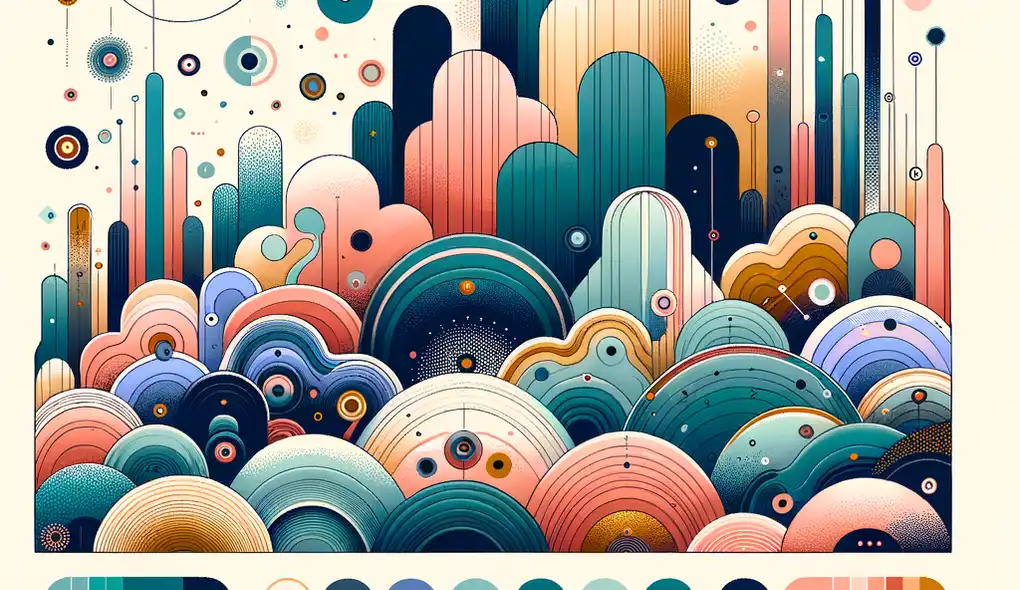
Quantitative research constitutes a foundational aspect of evidence-based decision-making in numerous scientific and business domains. It involves the systematic empirical investigation of observable phenomena via statistical, mathematical, or computational techniques. The primary goal of quantitative research is to establish patterns, understand relationships, and develop predictions about a particular subject. However, as one delves deeper into the field, several advanced topics emerge that challenge the traditional bounds of quantitative analysis, offering new dimensions of insight and innovative methods to tackle complex questions. This article aims to explore these advanced topics that can significantly enhance your expertise and value in the field of quantitative research.
Statistical Modeling Beyond the Basics
While most are familiar with linear regression and simple analytical methods, advanced statistical modeling includes techniques like multilevel models, structural equation modeling (SEM), and time series analysis, among others. Multilevel models allow researchers to take into account the hierarchical structure of data, such as students nested within schools, thereby improving the accuracy of estimates. Structural equation modeling combines factor analysis and multiple regression to understand complex relationships between observed and latent variables. Time series analysis involves techniques to analyze a sequence of data points collected over an interval of time, which is crucial in areas like finance and meteorology.
Novel Data Mining Techniques
Data mining is the process of discovering patterns and knowledge from a large set of data. As we enter the age of big data, advanced data mining techniques, such as cluster analysis, association rule learning, and neural networks, have become indispensable. Cluster analysis is used to segment populations or data sets into various groups with distinct characteristics. Association rule learning finds interesting relationships between variables in large databases. Neural networks, inspired by the human brain's architecture, are especially useful in modeling complex patterns and making predictions based on vast datasets.
The Emergence of Machine Learning
Machine learning, a branch of artificial intelligence, focuses on the development of algorithms that can learn from and make predictions on data. It encompasses a variety of advanced methodologies such as supervised learning, unsupervised learning, and reinforcement learning. The application of these methods has revolutionized fields such as computer vision, natural language processing, and predictive analytics. Understanding the principles and proper application of machine learning techniques is becoming increasingly important for quant researchers in various sectors.
Causal Inference and Its Complexities
Causal inference is the process of drawing a conclusion about a causal connection based on the conditions of the occurrence of an effect. Advanced topics in causal inference include instrumental variables, regression discontinuity designs, and propensity score matching. These techniques are designed to overcome the limitations of observational data and provide robust ways to infer causality where randomization is not possible.
High-Dimensional Data Challenges
High-dimensional data refer to data sets with a large number of variables relative to the number of observations. This poses distinct statistical challenges such as the curse of dimensionality, which can lead to overfitting and spurious results. Advanced statistical techniques like dimensionality reduction, regularization methods, and high-dimensional probability theory are crucial for making valid inferences from high-dimensional data.
Simulation-Based Estimation Techniques
Simulation-based estimation techniques like bootstrapping, Monte Carlo simulations, and Markov Chain Monte Carlo (MCMC) methods introduce robust alternatives to traditional estimation methods, especially in situations where analytical solutions are intractable or do not exist. Using random sampling and computational power, these techniques allow for approximating complex probability distributions and making inferences about population parameters.
Integrating Qualitative Data
Quantitative research is often criticized for neglecting the rich context that qualitative data can provide. Advanced quantitative researchers are now exploring methods to integrate qualitative data into their analyses. Techniques such as mixed methods research, where qualitative and quantitative data are combined, or qualitative comparative analysis (QCA), offer nuanced insights that purely quantitative methods may overlook.
Ethics and Responsible Analytics
As quantitive researchers gain access to more sensitive and comprehensive data sets, the need for ethical considerations and responsible analytics becomes paramount. Advanced discussions in the field revolve around data privacy, algorithmic bias, and ethical implications of automated decision-making systems. Researchers must be equipped with the awareness and tools to address these challenges effectively.
Future Trends in Quantitative Research
The future of quantitative research is being shaped by advances in computational power, the rise of open-source statistical software, and the ever-increasing availability of data. Keeping abreast of emerging trends such as quantum computing, edge analytics, and federated learning is essential for future-proofing one's skills and understanding their impact on quantitative research methods.
In conclusion, advancing your expertise in quantitative research involves not only mastering mathematical and statistical techniques but also exploring these sophisticated topics. Combining technical proficiency with an awareness of the ethical and practical complexities of the field ensures that researchers can contribute valuable insights and foster responsible, data-driven decision-making in their respective domains.
Frequently Asked Questions
Frequently Asked Questions
1. What are the key differences between quantitative and qualitative research?
Quantitative research focuses on numerical data and statistical analysis to understand relationships and patterns, while qualitative research delves into the deeper meanings and contexts behind phenomena through non-numeric data such as words and images.
2. How can I enhance my skills in statistical modeling?
To improve your skills in statistical modeling, consider taking advanced courses in multilevel modeling, structural equation modeling, and time series analysis. Practice working with real-world data sets to grasp the application of these techniques.
3. What are the practical applications of machine learning in quantitative research?
Machine learning techniques are widely used in quantitative research for tasks like predictive modeling, pattern recognition, and clustering. Understanding how to implement supervised, unsupervised, and reinforcement learning algorithms can significantly enhance your analysis capabilities.
4. How important is ethical consideration in quantitative research?
Ethical considerations are paramount in quantitative research, especially with the increasing volume of data and potential for bias in algorithms. Researchers must prioritize ethical practices, ensure data privacy, and address algorithmic biases to uphold the integrity of their research.
5. What are some emerging trends in quantitative research to watch out for?
Keeping an eye on trends like quantum computing, edge analytics, and federated learning can provide insights into the future direction of quantitative research. Adapting to technological advancements and new methodologies is essential to stay ahead in the field.
6. How can I effectively integrate qualitative data into my quantitative research?
To integrate qualitative data into quantitative research, explore methods like mixed methods research and qualitative comparative analysis (QCA). These approaches allow for a holistic understanding of phenomena by combining the strengths of both qualitative and quantitative data.
7. What challenges are associated with high-dimensional data in quantitative research?
High-dimensional data pose challenges such as overfitting, spurious results, and the curse of dimensionality. Utilizing techniques like dimensionality reduction and regularization methods can help mitigate these challenges and ensure valid inferences from complex data sets.
Resources
Further Resources
For those looking to delve deeper into advanced topics in quantitative research, there are numerous resources available to enhance your knowledge and skillset. Here are some recommended readings, courses, and tools to further enrich your understanding:
Books
- "Multilevel Analysis: Techniques and Applications, Third Edition" by Joop J. Hox, Mirjam Moerbeek, and Rens van de Schoot
- "Structural Equation Modeling: Foundations and Extensions" by George A. Marcoulides and Roderick P. McDonald
- "Pattern Recognition and Machine Learning" by Christopher M. Bishop
Online Courses
- Coursera: Machine Learning
- edX: Causal Diagrams: Draw Your Assumptions Before Your Conclusions
- Udemy: Data Mining and Machine Learning Techniques
Tools & Software
- R Programming Language: Official Website
- Python for Data Science: Anaconda Distribution
- IBM SPSS Statistics: IBM Product Page
Journals & Publications
- Journal of Machine Learning Research - Website
- Journal of Causal Inference - Submission Guidelines
- Quantitative Methods for Psychology - APA Journal
Webinars & Conferences
- Data Science Central: Upcoming Webinars
- International Conference on Machine Learning (ICML) - Official Website
- Quantitative Research Methods Workshop - Event Details
Associations & Communities
- American Statistical Association (ASA) - Website
- Society for Industrial and Applied Mathematics (SIAM) - Community Page
- Reddit Data Science Community: r/datascience
Data Repositories
- UCI Machine Learning Repository - Dataset Archive
- Kaggle Datasets: Explore Datasets
- Google Dataset Search - Research Data Discovery
These resources cover a wide range of topics from statistical modeling and machine learning to ethics in data analytics. By immersing yourself in these materials and engaging with the broader quantitative research community, you can stay at the forefront of advancements in the field and continue to hone your expertise in quantitative analysis.